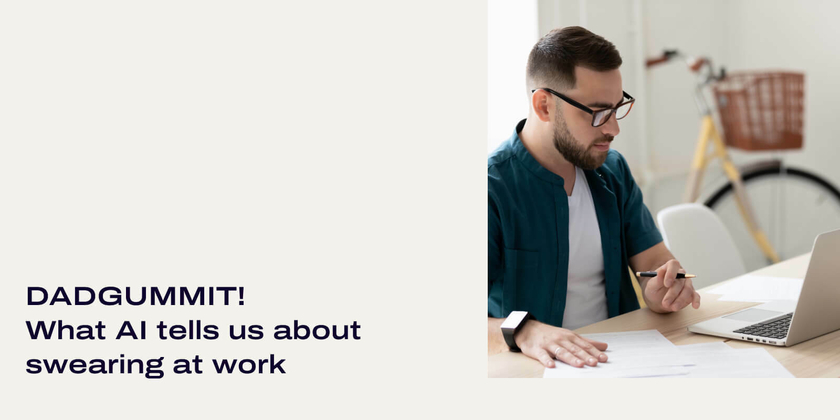
May 05, 2021
This is not a post about whether it’s ok to swear at work.
That’s been done to death, and moreover (a) it’s clear that who “gets to swear” is a question laden with social and institutional power dynamics, and (b) our data clearly show that people do swear on work related calls and videoconferences; profanity shows up everywhere in the call transcripts that Dialpad Ai generates on any AI-enabled call.
In fact, in the past year, almost 1 in 20 calls made (across all our products) included some form of profanity (i.e. one of the words from an internal list curated by our staff linguists).
Num of Swear words per 100 calls | Num of Swear words per hour | Percentage of calls with at least one swear word |
8.53 | 0.95 | 4.99% |
Table 1: Overall distribution of swear words in Dialpad Ai-enabled Dialpad calls
(here and below aggregated from Feb 2020 to Feb 2021)
By proportion, the top two or three swear words are very over-represented (although, see below for a potential confound in the case of sh*t), and seven of the "top 10" swears are alternative forms of the top 2 words.
We English speakers are apparently hesitant to leverage our linguistic creativity when it comes to profanity, at least when it comes to business conversations.
Top 10 swear words | Ratio (percentage) |
sh*t | 39.49 |
f*cking | 29.56 |
f*ck | 13.03 |
*ss | 5.07 |
bullsh*t | 4.1 |
f*cked | 1.97 |
sh*tty | 1.96 |
b*tch | 1.04 |
*sshole | 0.63 |
f*cker | 0.52 |
Table 2: Top 10 swears by frequency
Considered in isolation, an instance of profanity doesn’t provide much information. It’s well-known that context is highly determinative of the perceived impact and appropriateness of workplace profanity. One-on-one peer meetings are different from a sales call (learn more about sales call reporting), which is different from a customer service interaction, or a team brainstorming session. Drilling down a bit into the above statistic, a more granular picture of swearing in the workplace emerges.
What the hell does the data tell us?
A quick refresher, in a Dialpad context:
We have a general phone calling app—Dialpad Talk (now known as Dialpad Ai Voice)
We also have a sales dialer tool—Dialpad Ai Sales
There's the cloud contact center solution—Dialpad Ai Contact Center
And even video conferencing—UberConference (now known as Dialpad Ai Meetings)
Immediately it becomes clear that the different types of calls that occur across these platforms reveal different social conventions concerning the acceptability of profanity across different business contexts.
Dialpad Product | # of swear words per hour |
Ai Contact Center | 0.47 |
UberConference | 0.83 |
Talk | 1.62 |
Ai Sales | 0.80 |
Table 3: Swear words distribution for each product
What does this mean for our customers? Well, if I manage a call center, being alerted when a swear word shows up in a call could be a sign that something has gone awry and that I might need to intervene.
Conversely, on a sales call, it could be a sign that a good interpersonal rapport has been established and that the call is in fact going well, or it could be an example of a sales person using mirroring to try and foster trust!
In any of these situations, what remains true is that we need to render faithful transcriptions of the call contents, so that contextually appropriate action may be taken.
We've looked at how trends in swearing differ based on the type of conversation being had, now let's look at how those trends may change over time.
There are a couple points of interest in the timeline shown below:
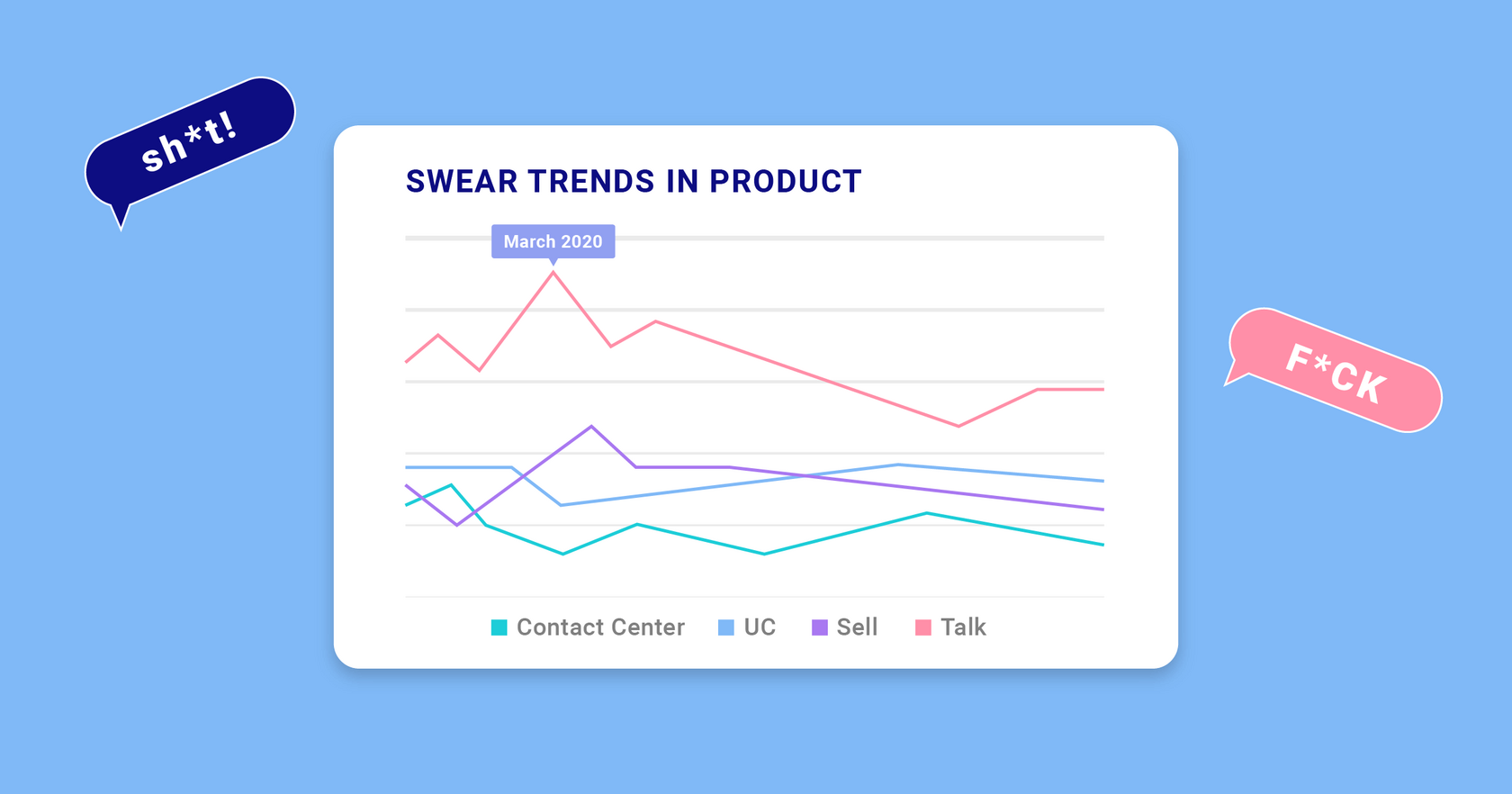
As one would expect from the aggregate data in Table 3, the topmost line in Figure 1 is from Talk, the bottom line is from Ai Contact Center, and the middle two are UberConference and Ai Sales.
One feature of interest is that in our customer support and sales-focused products, there were upward blips around March of 2020, right around the time when COVID started really entering the public consciousness and lockdowns and work-from-home orders began rolling out.
Again, we see the context-dependence of profanity; Talk and Ai Sales are both platforms where some degree of small-talk is likely to occur—that is, less formal or sociologically-restricted conversational contexts, and hence plausibly more opportunity to discuss world affairs.
Once again, a richer picture emerges if we drill down with a different point of view: trends for the top 5 swear words by frequency.
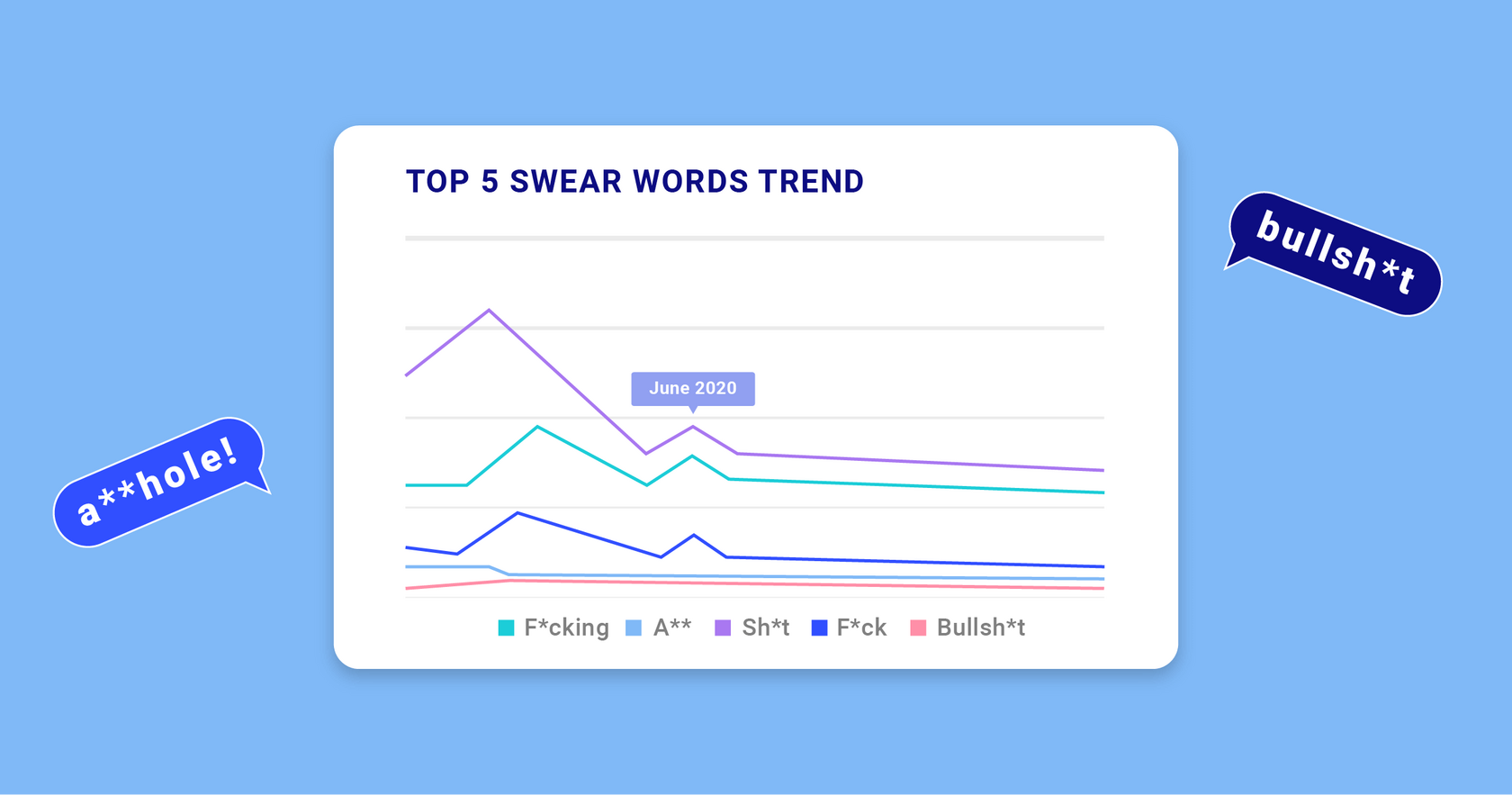
Again, we see a clear upward blip right around the time when news of COVID-19 and lockdowns began to spread in earnest.
In this case, there’s a sharp uptick and reversion to “normal” levels in instances of shit amidst an otherwise general downward trend, whereas the rises in fuck and its derivatives clearly took much longer to taper off; an analysis of our data shows that instances of fucking crazy took off around this period.
Curiously, there is another blip for the week leading up to June 28th, and we can see that shit and fuck(ing) are all “time-locked” in the sense that all three show a brief increase then reversion to previous levels. Although it’s difficult to point to any specific event causing this sharp rise in profanity, it was a busy week around the world, and it was also the week during which worldwide COVID-19 cases surpassed 10 million.
What the f**k? That’s not what I said!
Having seen the numbers above, you may wonder: "Since most of us use swear words to at least some extent in our daily conversations, and I can tell when someone is swearing immediately (or can I?), it should be easy for a speech recognition system to identify them, right?"
As a rule, the answer is “yes”. Given that swearing is common in conversation and our speech recognition system is trained on typical conversations, the model should be able to detect and recognize profanity easily, but of course it turns out that there’s a catch: an ASR system can be too sensitive.
This notion of being “too sensitive” gives rise to a challenging issue with respect to accurately transcribing profanity, namely so-called false positives, that is, situations in which profanity is transcribed when in fact none was uttered.
Shortly after I joined the ASR team at Dialpad, my manager pulled me into my very first 5 Whys meeting; one of our most important customers had contacted our CEO directly, upset because the transcripts of their work calls were full of swear words and they couldn’t understand why. Resolving the issue for them (in addition to finding out its cause) became a drop everything priority.
It is a truism among linguists that people are notoriously inaccurate when it comes to self-reporting their language use. After listening to a sampling of the client’s call audio, it became clear that a significant proportion of the profanity in their transcripts was being uttered on their calls (albeit neither more nor less than anyone else).
Nevertheless, the client preferred not to see any profanity in their call transcripts, and so as a short-term stop-gap we censored words from our internal list of profanity directly in the application interfaces, rather than via tweaking the ASR.
As it happened, our data dive had the fortuitous side effect of revealing that many swear words were showing up in call transcripts that were definitely not in the call audio recordings. We realized that there were some discernible patterns: the presence of what linguists call “broad-spectrum” noise (like when you make a shushing noise) often resulted in the word shit appearing in the transcript, and the word “fact” was often transcribed as some variant of fuck.
The cause of these types of mistranscriptions is simple enough to understand when you know the broad strokes of how an ASR system works: one part of the system, the acoustic model, predicts words on the basis of sounds (did the speaker say speech or beach), and a second part, the language model, tries to construct a plausible sentence from the predicted words.
(Did the speaker say recognize speech or wreck a nice beach? This is a very simplified description—stay tuned for our ASR 101 post for a deeper dive into the automatic transcription of speech to text).
All else being equal, broad-spectrum noise sounds just like the first sound in shit, and the word fact overlaps significantly in sounds with the word fuck. But that’s not enough on its own; the language model should still be able to correct for these types of mistakes (is it more likely that the caller said as a matter of fact or as a matter of fucked).
The problem lies with the data that was used to create the language model, which generates sequences of words; upon investigation, we found that one of the collections of texts we used to create the language model was made up of a series of “meeting” transcripts that contained a grossly disproportionate amount of swearing.
In fact, these were not meeting transcripts at all but rather voice chats between gamers, a speech community that is definitely known to be "linguistically creative" and plentiful in their use of profanity! We had inadvertently but rather effectively biased our own model towards swearing like a sailor!
Simply removing that one corpus from our collection of training data went a long way toward mitigating the presence of false positives in our transcripts.
Who gives a crap anyways?
Whether people like it or not, swearing is a common component of conversations. People may swear when they are frustrated or conversely swear to show their excitement (consider the difference between fuck you and fuck yeah)! Swearing isn't inherently bad or good, it depends on context and individual perceptions.
Not to mention understanding the contexts within which swearing is used can unlock important business insights for an organization, one of the major ones being the identification of angry customers. The desire to explore these insights has led us to allow customers to choose whether they would like to see swearing in their transcripts or not.
Recently, at the request of our customers, we’ve begun displaying profanity in our transcripts again, but now with the option for users to toggle whether or not swear words are displayed directly, or replaced with the identifying tag "[expletive]".
Why reinstate them? Swearing is a core component of conversation; people may swear when they are frustrated, or when they are happy, some people may use swearing to show their identity.
Crucially, swearing isn't always a bad thing, and in many cases it may be informative; giving Dialpad’s customers the tools to analyze and understand the contexts and prevalence of swearing in their calls can be a valuable source of business insight—which, ultimately is the reason that Dialpad uses AI in the first place.
References
Swearing at work
https://www.bbc.com/worklife/article/20160802-swearing-at-work-might-be-good-for-your-career
https://www.forbes.com/sites/davidsturt/2015/03/19/is-the-f-bomb-appropriate-at-your-work
https://qz.com/242637/the-complete-guide-to-swearing-at-work/
https://www.lexology.com/library/detail.aspx?g=62bcc2bb-ab2c-45f6-837f-cd7cdbdfc1e8
https://www.cnn.com/2019/07/22/success/swearing-at-work
Social and power dynamics
https://othersociologist.com/2018/09/16/sociology-of-swearing/
Context
https://link.springer.com/article/10.1007/s10936-014-9345-z
The prevalence of profanity
https://www.smithsonianmag.com/science-nature/science-swearing-180967874/
https://readable.com/blog/the-science-of-swearing/
Finally understand how transcribing conversations and calls can reveal insights that you didn’t know existed.
Try Dialpad for Free