NLP in customer service: How it’s used and where it’s going
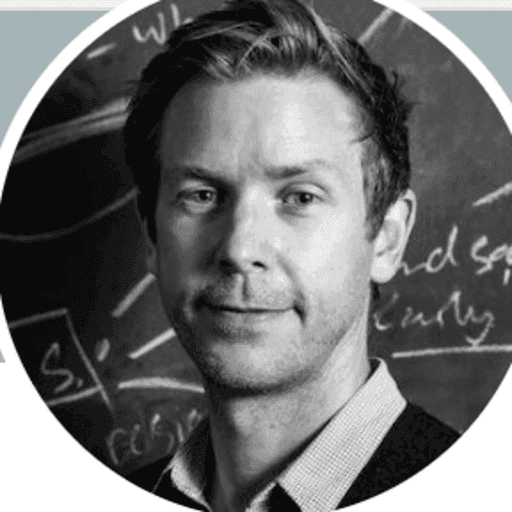
Senior Director, Product Management
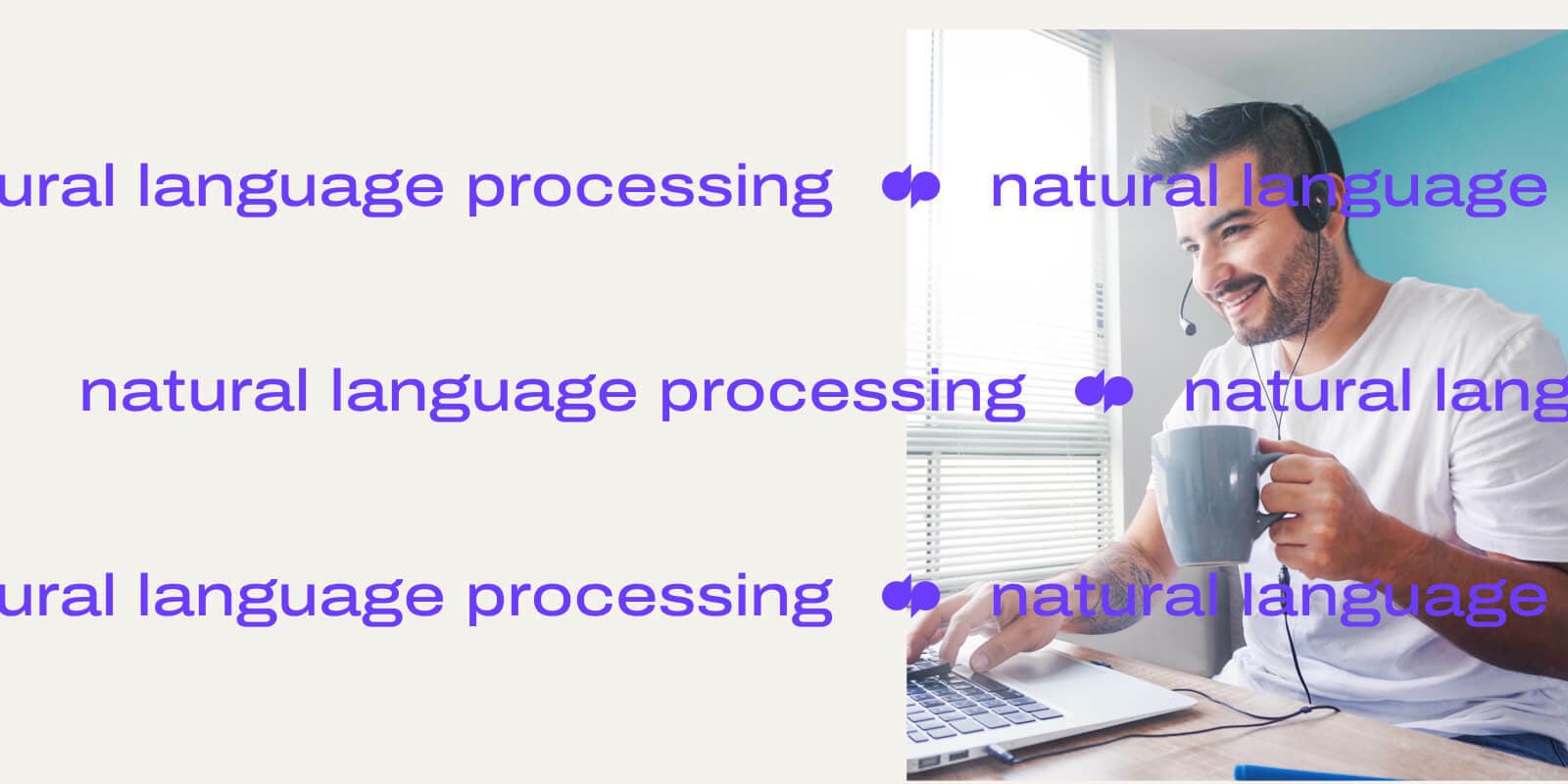
Tags
Share
Today, there’s a lot of talk about AI in customer service, but one piece of this puzzle that not many people talk about is NLP, or Natural Language Processing.
NLP is actually one of the most important tools when it comes to providing excellent customer service. At Dialpad, I lead a team of support agents, and we’re fortunate to be using our very own AI product that uses NLP to make agents’—and supervisors’— jobs easier.
In this blog, I’ll break down what NLP is, and how it’s already being used across the customer service experience today. (You’re probably already familiar with a few of these.)
Let’s dive in.
What is Natural Language Processing?
Natural Language Processing, or NLP, is a branch of artificial intelligence that deals with language-based interactions between computers and humans. Specifically, it’s meant to help computers understand human language—and respond in a way that is natural for humans.
At a high level, NLP works by ingesting data (in this case, written or spoken text), extracting the information and “learning” from that data, then making an educated guess as it generates an output (for example, answering a customer question).
Generally, you need AI experts and linguists to “teach” the NLP engine things like grammar and syntax, and how to recognize the subject or object of a sentence. Otherwise, it can’t “understand” what a customer means when they say, “I can’t log into my account” and provide the answer for that customer. (And as we all know, there are synonyms, homophones, and the fact that many grammar rules in English have exceptions.)
👉 Did you know?
Not all NLP models are made the same! The quality of NLP models can vary greatly depending on their inputs—different data in, different quality out. Google Bard and OpenAi’s ChatGPT, for example, use two different NLP models known as Large Language Models (LLM), but with different data. Dialpad Ai, for example, uses a huge proprietary data set that’s specific to business communications. This makes its outputs very specific to the contact center use case—and along with its ease of use in design and in-house team’s (including many PhDs!) leading AI expertise—makes Dialpad a uniquely powerful AI communications platform for businesses.
If you’re familiar with generative AI tools, like ChatGPT, you’ve already seen NLP in action. Having processes large amounts of natural language data, these tools can then “answer” any questions or inputs that you enter, and what seems to have impressed people most is how natural those responses sound. That’s the magic of NLP.
How is NLP used in customer service?
NLP is the basis for any “smart” service or AI that analyzes phone calls or messaging conversations for customer service teams. It can be used in a variety of ways in customer service, from improving customer-facing interactions with real-time coaching for agents, to gathering customer insights, analyzing customer sentiment, and more. Essentially, wherever there is spoken or written language in your customer service journey, NLP plays a role.
We’ll look at a few of these customer service use cases where NLP is used in more detail in the next section, but there are numerous possibilities—and it’s still early days!
5 use cases of NLP in customer service
1. Analyzing customer sentiment
When supervisors are overseeing agents, they might have tens or even hundreds of live customer calls happening simultaneously. How can one person monitor everything?
One thing NLP can do is provide conversation analytics, such as by flagging sentiment in these calls based on the words being used in the conversations.
Whether it's a price objection, a satisfied customer, or a general lack of enthusiasm—NLP can pick up on certain words or phrases to understand the customer’s sentiment. If it’s positive, that’s great!
For example, this is what Dialpad Ai does:
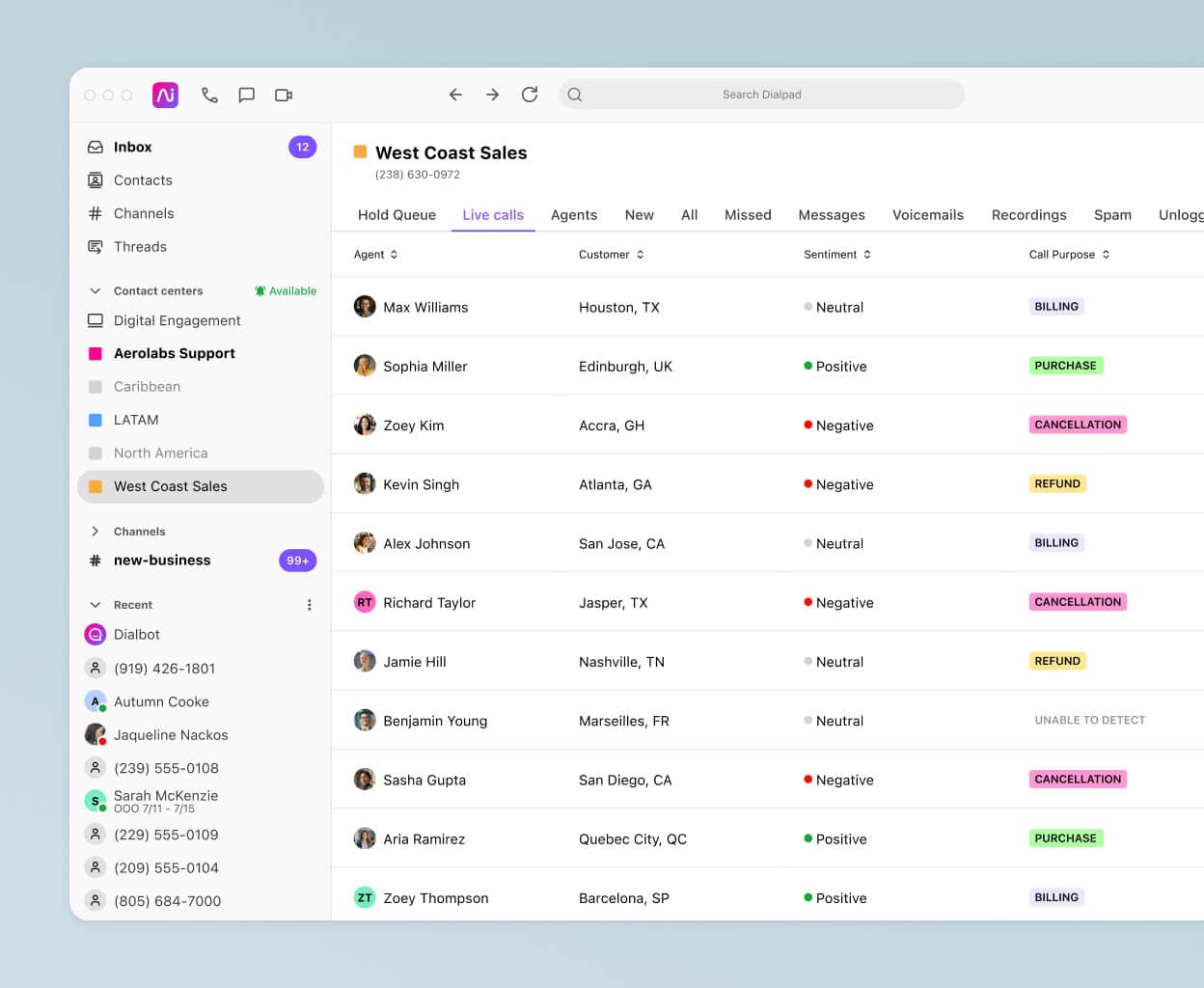
If a supervisor sees a call with negative sentiment, they can quickly pull up the real-time transcript to get more context before deciding if they need to listen in or barge the call to help de-escalate.
For example: If you get these responses from feedback forms:
“The agent I spoke to was awesome.”
“My order arrived quicker than I expected.”
“It’s easy to sync my data. Thanks for putting together your onboarding docs!”
The machine learning system will then tell you that the vast majority of feedback is positive. This gives you a rough understanding of how well you’re performing.
📚 Further reading:
Learn more about how we built this sentiment model in Dialpad Ai.
2. Conversational AI
Many people use “chatbots” and “conversational AI” interchangeably, but they’re technically two different things. Yes, both chatbots and conversational AI do similar jobs—they’re usually placed on a website to answer customer questions automatically to remove some of the burden from live agents.
But conversational AI is different in that it’s a more advanced form of chatbot. Whereas chatbots typically can only answer preset questions with preset answers, conversational AI is much closer to being able to simulate a conversation with a customer.
For example, see how in this example on Rogers (a telecom and internet provider in Canada), the customer says they need to set up a move for their services instead of selecting one of the preset options, and the AI understood, thanks to NLP—and provided the correct answer options:
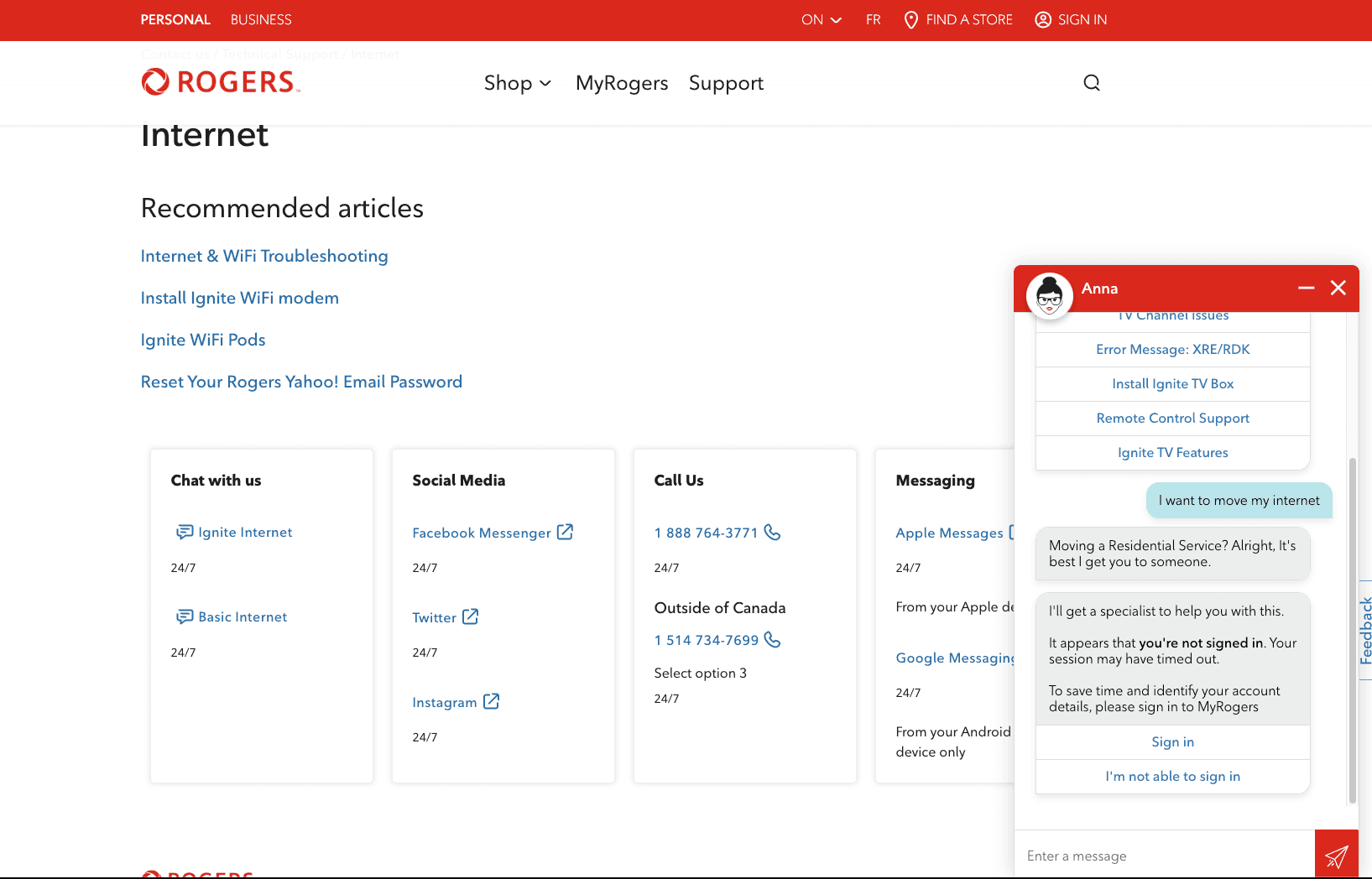
A traditional chatbot would not be able to do that.
This is exactly what Dialpad’s Ai Virtual Assistant can do. A contact center manager can set up conversational flows in just a few clicks with the drag-and-drop builder, no coding needed:
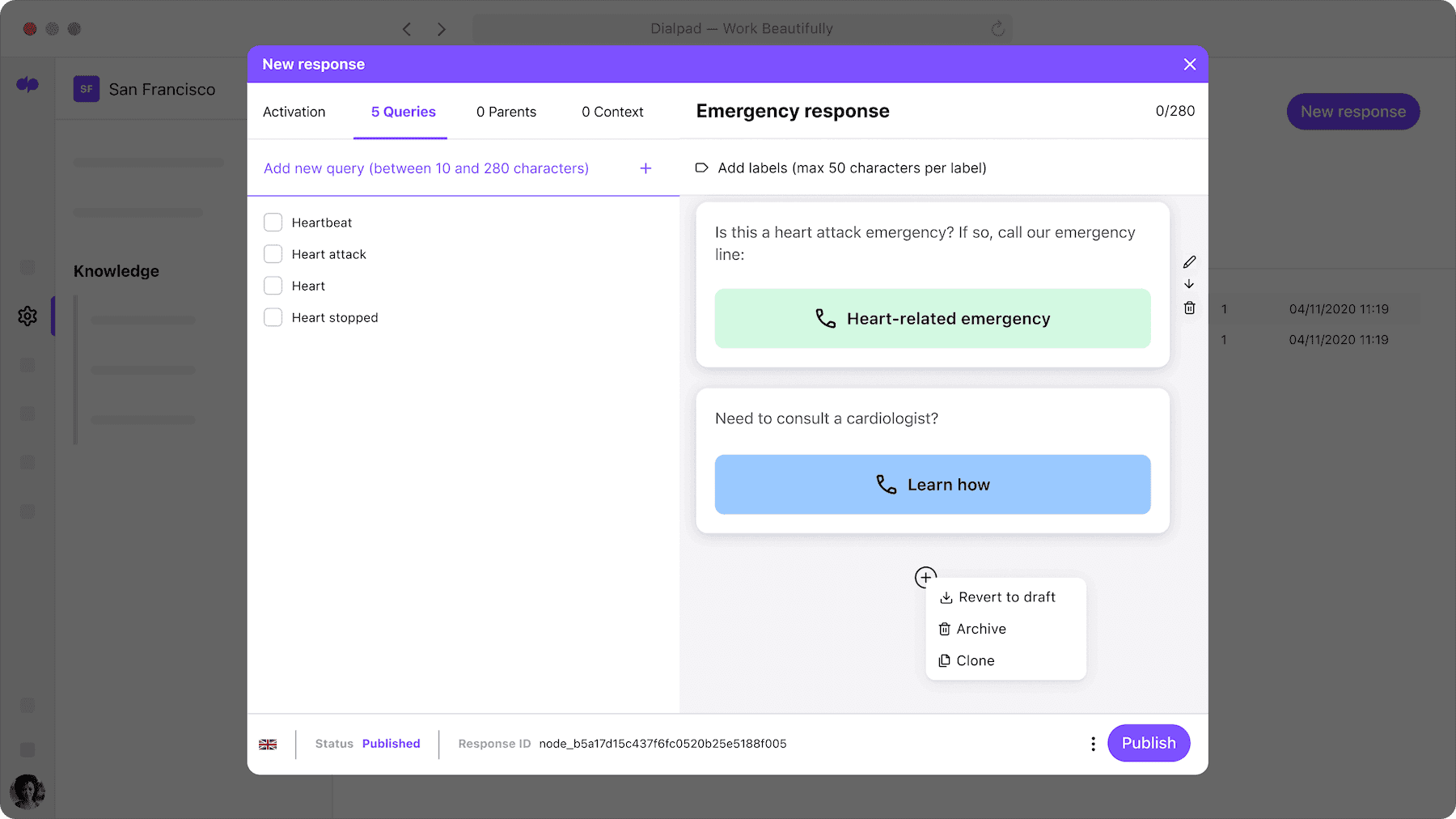
And more importantly, they can build in escalation options for customers so that if the AI is unable to answer a particularly tricky question, the customer can transfer the chat to a human with just a click.
Speaking of which…
3. Live agent support
NLP doesn’t just improve the customer experience, it can also help your customer support agents live during conversations.
We already mentioned that conversational AI can help deflect calls and reduce overwhelming volumes of inquiries for agents.
But beyond that, NLP can also play a part in AI-powered real-time assists for agents. For example, Dialpad’s Ai Agent Assist can understand customer questions and automatically search all connected knowledge sources (even unstructured sources like PDFs and past customer conversations) to find helpful information for agents in real time:
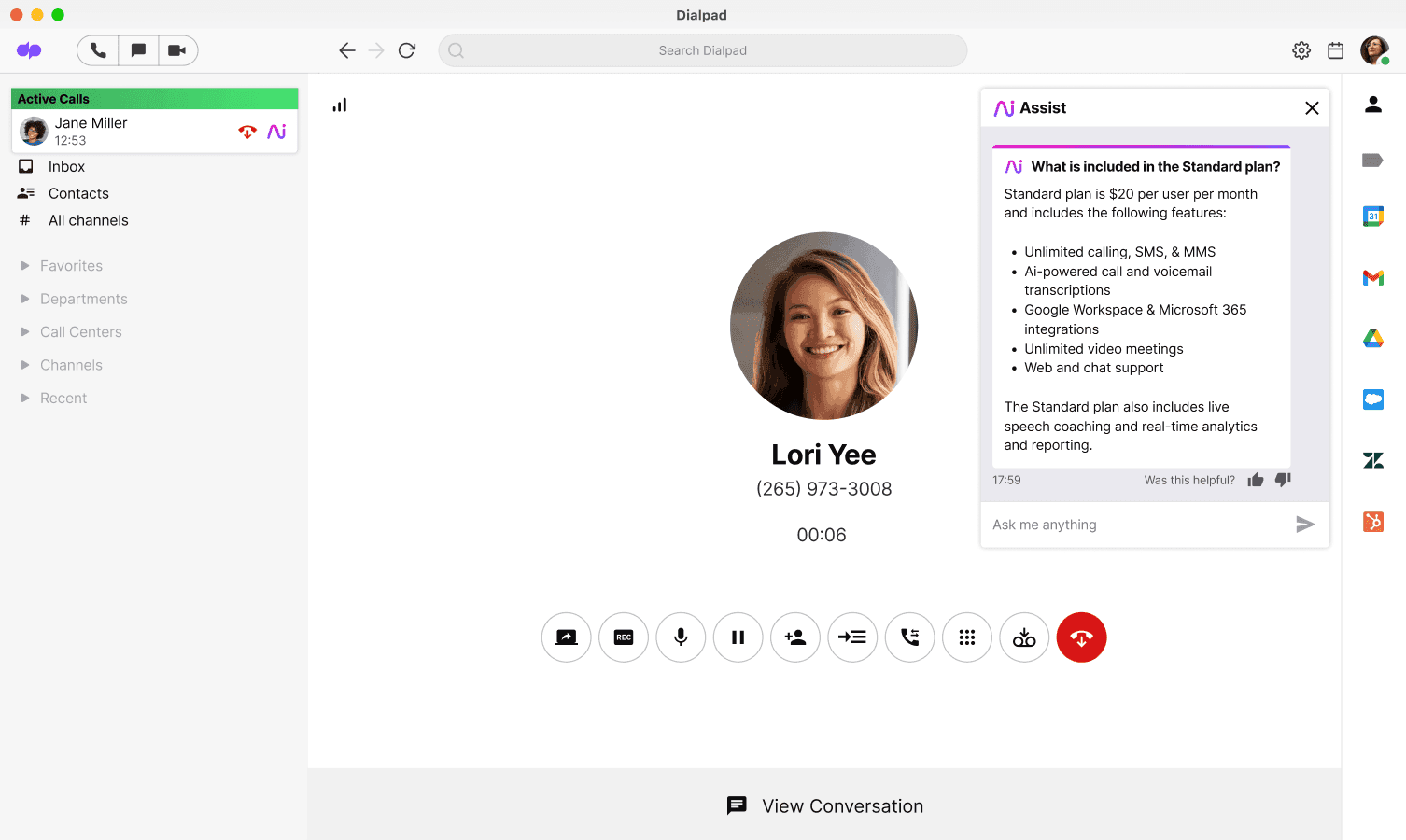
All without the supervisor having to lift a finger.
4. Improve training and knowledge base
On a related note, contact center AI can also use NLP to fill in gaps in training and knowledge bases.
This all depends on the robustness of the AI and NLP engine—some customer service software that claim to have AI may not be able to do this at all. But how it essentially works, at least with Dialpad Ai, is that the AI can flag where there’s missing content to supervisors when customers ask questions that aren’t covered in the current knowledge bases.
This automates a lot of that ongoing maintenance work when it comes to updating contact center training materials, and can save supervisors and enablement teams quite a bit of time.
5. Capturing conversation insights
NLP can also help with capturing insights from conversations.
Dialpad Ai, for example, can capture key moments and action items during calls and video meetings, which are automatically emailed along with a searchable transcript in a post-call summary to attendees:
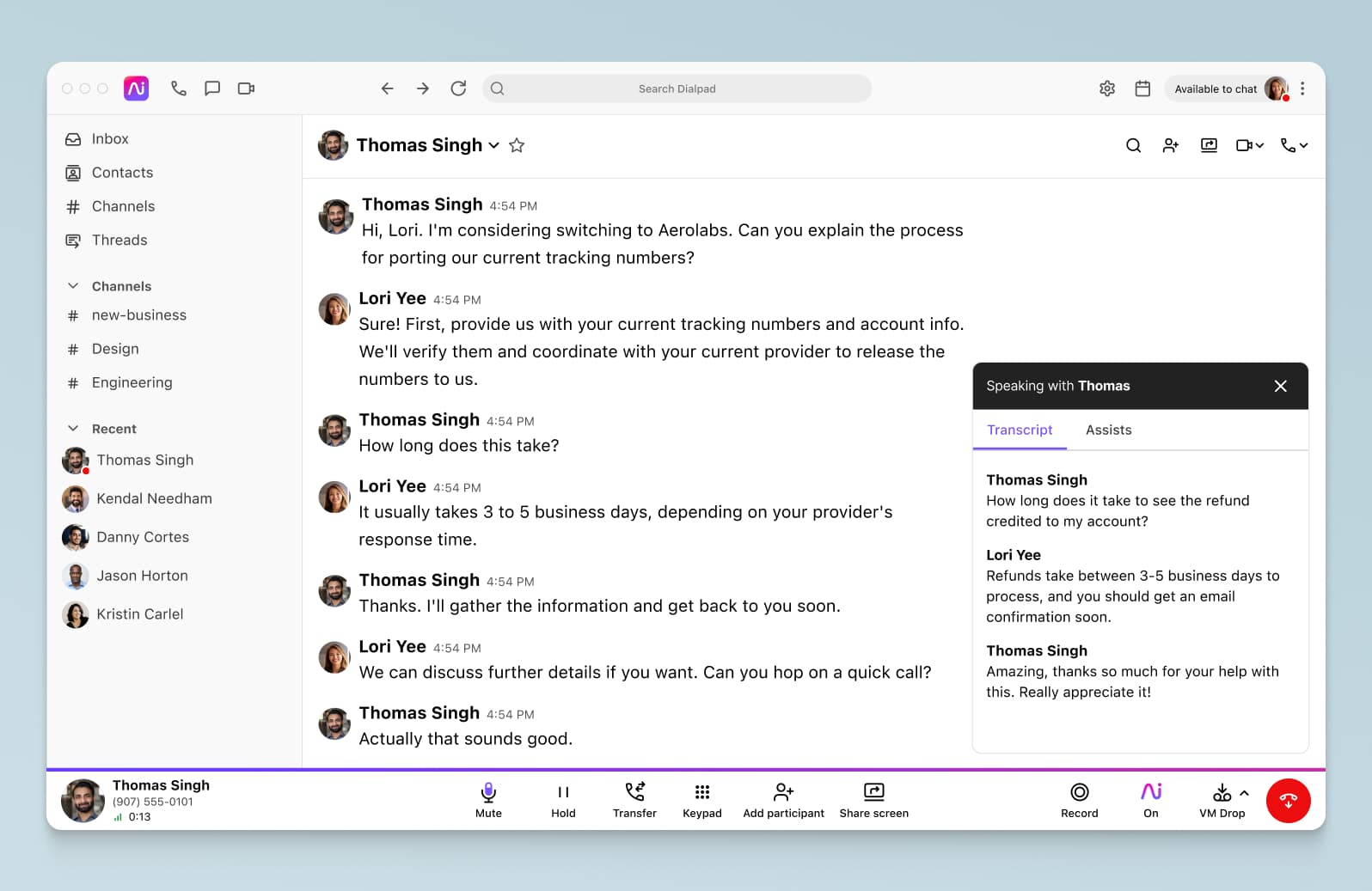
This can be helpful for supervisors and agents who may need to double back and review certain customer calls. But alongside this, supervisors can also track how frequently certain topics come up in customer support conversations.
For example, if they want to see how often customers are calling in about refunds, they can create a “Custom Moment” in Dialpad to monitor how often “refund,” “cancel,” and/or “money back” is mentioned on a call:
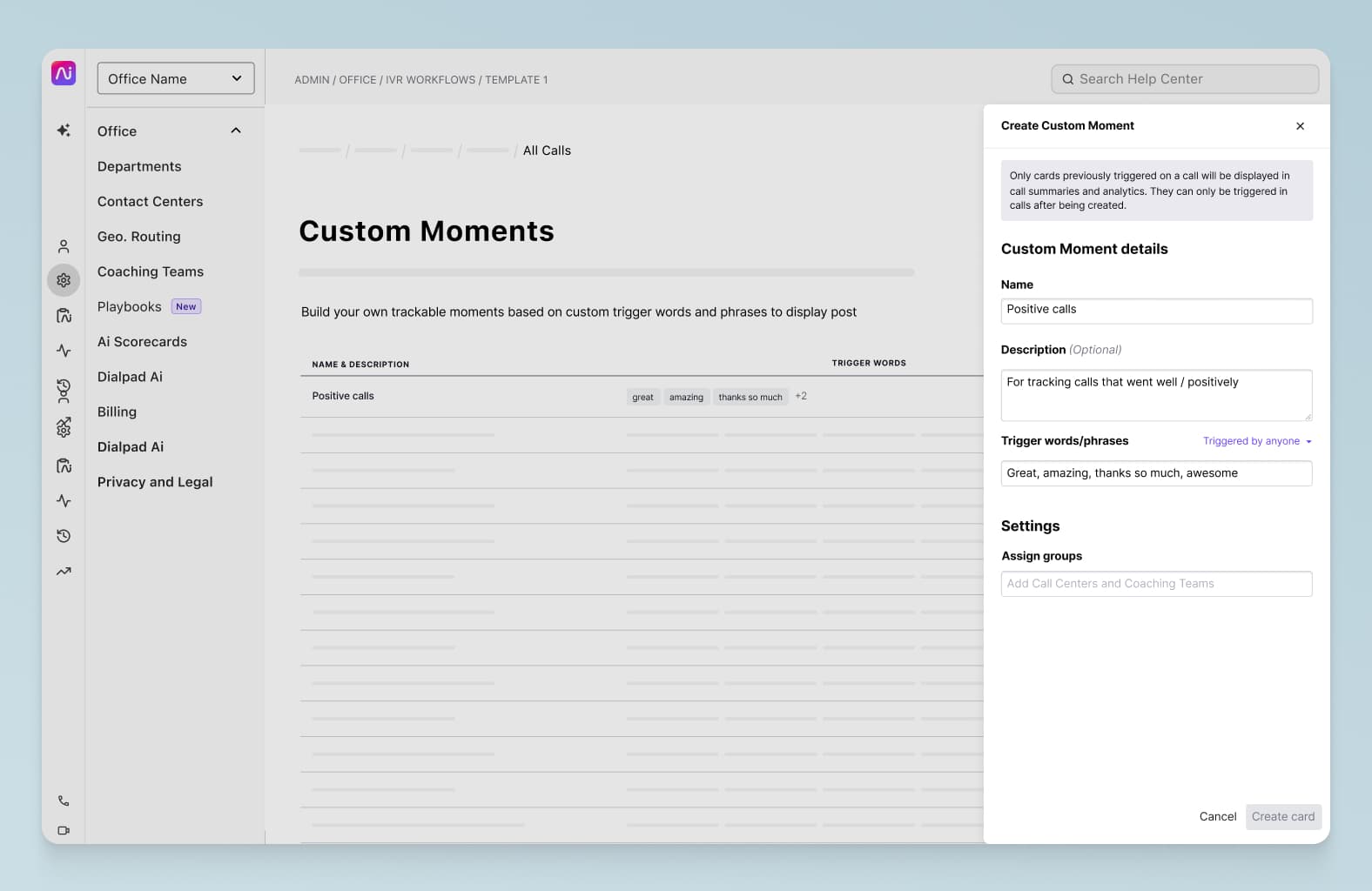
You can track pretty much anything with Custom Moments—dates, email addresses, frequent support issues, product names—and yes, even curse words—can be tracked and set up with alerts.
But besides this, NLP can help with other post-call tasks as well. Because NLP processes language and conversations, it can even do things like suggest dispositions for calls to reduce after-call work (ACW) time for agents and help automate QA scoring, which saves time for supervisors.
Many people still only think of voice transcriptions when they hear “capturing conversational insights,” but the possibilities really are endless, and we’re only just starting to see what customer service teams can really do with NLP.
The benefits of NLP in customer service
As we touched on earlier, there are more than a few benefits to using NLP in customer service. Let’s dig into each of these a bit more.
An improved customer experience
One of the biggest and most commonly cited benefits of using NLP in customer care is its ability to improve your overall customer experience.
While it doesn’t directly make agents better at their jobs, it does reflect the needs of today’s consumers much more than traditional phone support without AI.
Customers want answers now, on the channels they prefer, and they want the freedom to do it on their own schedules.
NLP-powered self-service AI and other automated channels like online FAQs, knowledge bases, and conversational AI can give your customers the answers they need, 24/7.
More productive agents
Smart companies aren’t planning to use AI to fully replace their human agents. They understand that customers will always require some degree of human touch, and are using AI and NLP to find ways to make their agents better at their jobs and ultimately provide a higher level of customer service.
From real-time agent assists, to live supervisor support, to updating training materials, there are endless ways to use NLP in customer care to empower your teams. (Learn more about how AI is transforming call centers.)
In fact, in our State of AI in Customer Service Report, we found that contact centers using Dialpad Ai reduced average call duration by 10.06% and lowered abandon rates by 17.36%.
A national car rental company using Dialpad Ai in its contact center found that its agents took about 50% more calls with the help of AI:
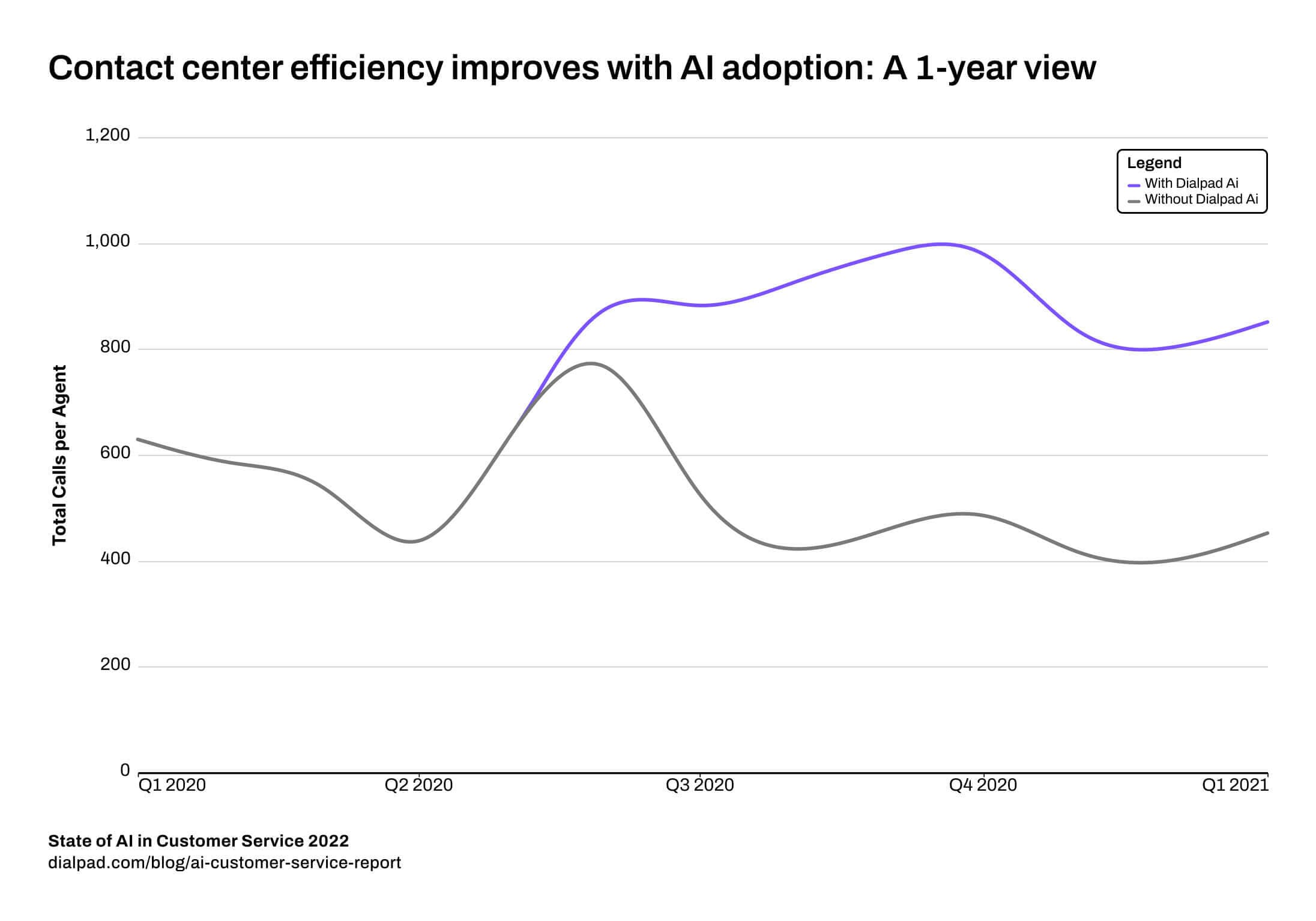
NLP can help gather valuable business data
Last but not least, one of the most powerful ways that customer service teams can use NLP is to analyze data and report on performance.
Companies’ most valuable sources of insights live in their everyday conversations with customers. Every single day, customers are telling you what they like about your products and services, what they don’t like, where the issues and gaps are, and more—the hard part is trawling through all the phone call recordings, social media messages, live chat messages, and service tickets.
NLP can help with that.
Beyond tracking keywords and topics, which we talked about above, NLP can also help sort and analyze data related to your contact center KPIs.
For example, one of the biggest challenges with gathering CSAT scores is that not a lot of people actually fill out those surveys. In fact (depending on the industry and specific business of course), we've found that on average only about 5% of customers actually fill out CSAT surveys.
On a related note, usually only the angriest—and happiest—customers actually bother to respond to these surveys, which means your CSAT answers are likely to be very skewed and not representative of how your customers feel overall.
Dialpad's industry-first Ai CSAT feature is designed to solve exactly that. Not only can Dialpad Ai transcribe calls and analyze sentiment in real time with NLP, it can also infer CSAT scores for 100% of your customer calls.
The result: a much more representative sample size for CSAT scores, and a more accurate understanding of how satisfied your customers really are:
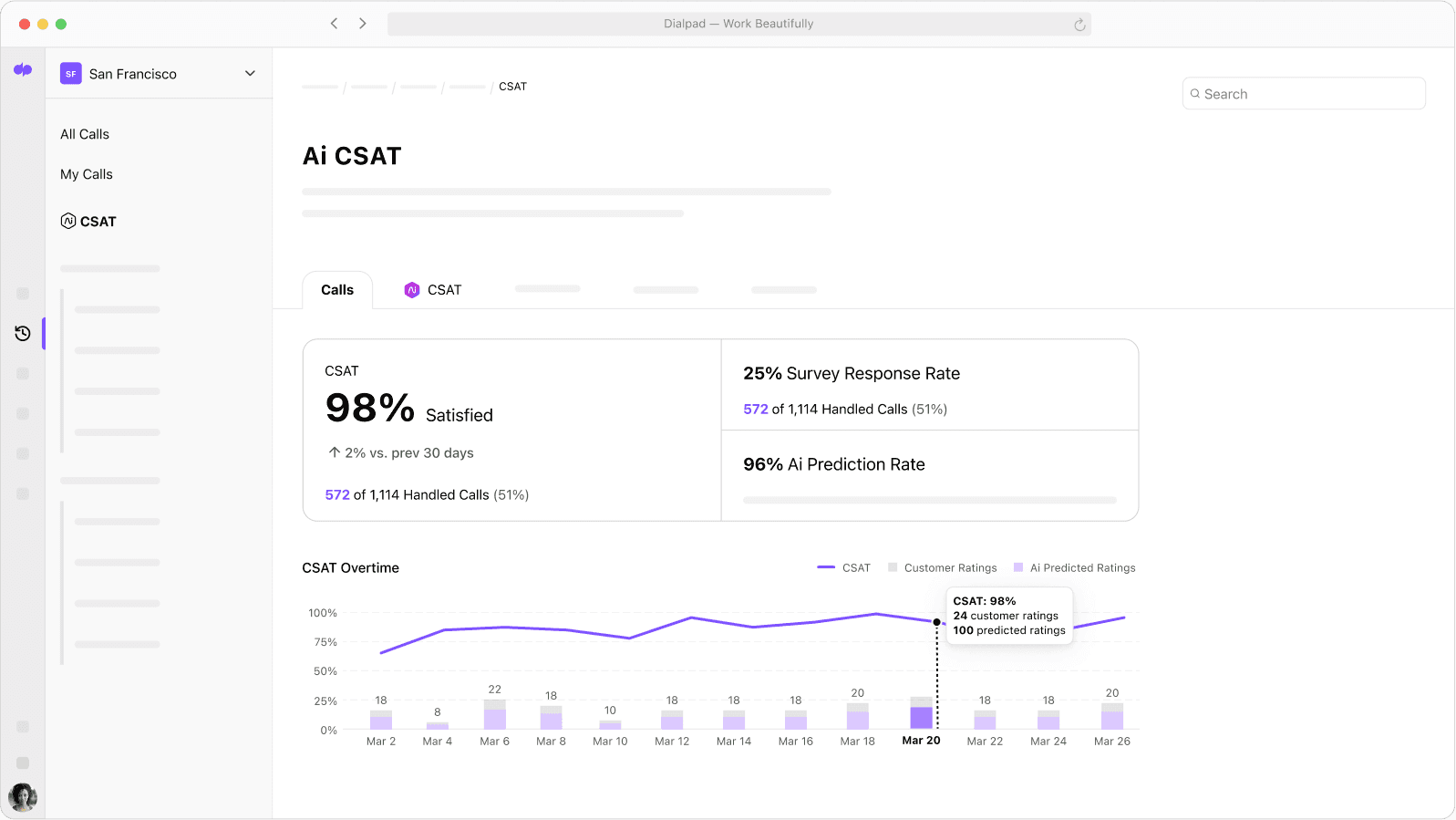
Are you using NLP in customer service?
Even though it’s early days, the data is clear—companies that are using AI and NLP in customer service aren’t just seeing a measurable improvement in performance from their agents.
Their supervisors are more productive and able to glean insights more easily, and their business leaders are able to make more strategic, data-informed decisions using both qualitative and quantitative feedback from their customers.
If you’re curious about how your teams can use Natural Language Processing, get in touch to see how Dialpad Ai Contact Center works—and what makes its unique proprietary AI different from other AI solutions!
Get a hands-on look at NLP in customer service
Book a demo to see how it works in Dialpad Ai Contact Center, or take a self-guided interactive tour of the app on your own!