Here are some of the tools we think you’ll be using a few months (or years) from now.
We're living in the Bronze Age of artificial intelligence.
Today’s generative Ai tools are vastly superior to their predecessors and have made Ai truly useful to new audiences of hundreds of millions of people all over the world. But even as research is expanding in every direction—computational linguistics, multimodal agents, reasoning, alignment, and safety, to name just a few—it’s still early days, and some of the most promising uses remain challenging and out of reach for today’s Ai.
Maybe in the next few years a grand unified theory of Ai will emerge. For now we have thousands of startups building and selling Bronze Age tools and working to imagine a golden future whose technology has caught up to our newly raised ambitions.
Dialpad embraced Ai in 2018 and we’re still the only Ai-native business communications platform. As GenAi transforms customer service and business collaboration, here’s a look at some of the more speculative stuff we’re working on as we continue to build the future of business communications.
Customer knowledge
Delighting customers by having the answer ready before they even ask their question? That's 9 billion minutes of experience talking.
Understand the state of play with DialpadGPT across all features
We built DialpadGPT specifically to do Ai Recaps. But these models need to do more than just summarize conversations; they need to answer agents’ questions about ongoing conversations. We’re retraining DialpadGPT on business calls and analytics and planning to migrate over features like Ai Playbooks and Ai Scorecards from third party LLMs. And beyond that, we’re looking at answering ad hoc questions like, ‘What was the customer's address?’ or ‘What was discussed in this meeting about headcount?’ From there, we see our GPT summarizing issues from multiple conversations and, ultimately, being capable of wielding language and other information in order to answer questions like, ‘How many customers called in today with negative sentiment?’ or ‘What are the top five topics occurring in calls over five minutes long?’
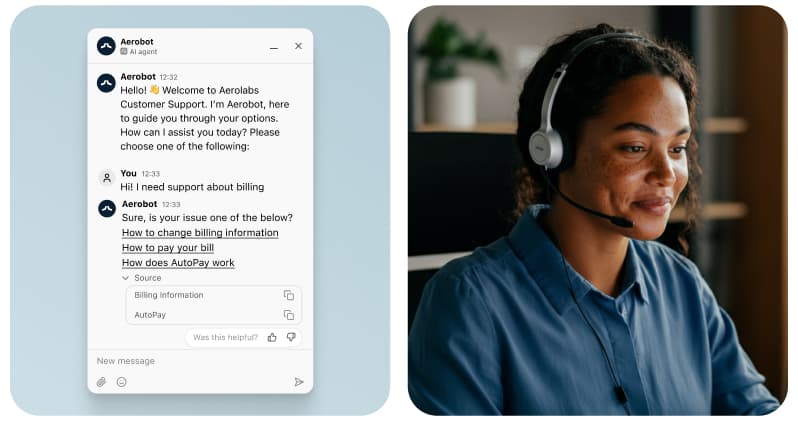
Answer questions once and well by mining Q&A
When a customer asks a human agent a question, we want our Ai to be able to feed the agent the answer in real time. The current Dialpad chatbot is trained on a company’s knowledge base. But not many companies have comprehensive knowledge bases that cover all the information that’s captured in call transcripts. Our first step in solving that problem will be to study the transcripts of historical conversations and use signals like call disposition—which quickly categorizes the call’s outcome—to create a database of frequent questions and answers. This sets the stage for a kind of coaching, where the model can help human agents by surfacing correct answers when calls don’t go well. Ultimately, we’ll be able to extract all kinds of data from historical transcripts and understand it well enough that our chatbots become fully effective self-service agents.
Instant improvement with proactive recommendations
Features like Ai Playbooks and Ai Scorecards help Dialpad customers better understand their customer interactions. But setting up these tools can be tricky; today we ask you to choose from a list of potentially relevant questions and topics or list your own questions,
a potentially powerful tactic whose success is difficult to predict in advance. So we’re going to make this process a lot easier. For cold-start customers who have no data and no idea how to begin, we’ll offer a starter kit of industry-specific topics. When companies do have data, we can suggest questions that track the topics we see being discussed. And once we’re thinking about recommendations, there’s no end of services they could
improve; Ai agents and Ai Assistant, for instance, could both be more powerful with this kind of data. Frankly, we could sprinkle recommendations almost anywhere on the platform. Wherever you are on Dialpad, you should have instant access to the best ideas and insights your Ai models have to offer.
Post-call insights
Just as your Ai tools should empower your teams in advance, they should also deliver more detailed insightful feedback after calls and meetings are over.
Automate dispositions to provide insights about all customer calls
It's important to be able to track information about your contact center’s call outcomes. Unfortunately, many companies barely tackle this difficult problem today. Only a tiny fraction of these customer calls actually have an agent assigning a disposition afterward; in most cases, you can’t even tell after a given call whether the issue was resolved, let alone any other annotations that reflect how the conversation went. Later this year we
plan to roll out an automation that can tag every single call with a phrase that can help for all kinds of analysis such as studying whether specific phrasings or flows lead to the successful resolution of customer issues.
Make every meeting your meeting with interactive summaries
When you finish a meeting, it’s nice to have an immediate summary of what was discussed. Dialpad delivers that today, but it’s static–everyone on the call gets the same summary, and all you can really do is vary the document’s length or format. As a next step, we plan to add topics as part of the summary and each participant can choose a summary tailored to one of those topics. And eventually, we’ll be able to offer ad hoc summaries with any emphases you request, or discussions of a topic from multiple meetings combined in one summary.
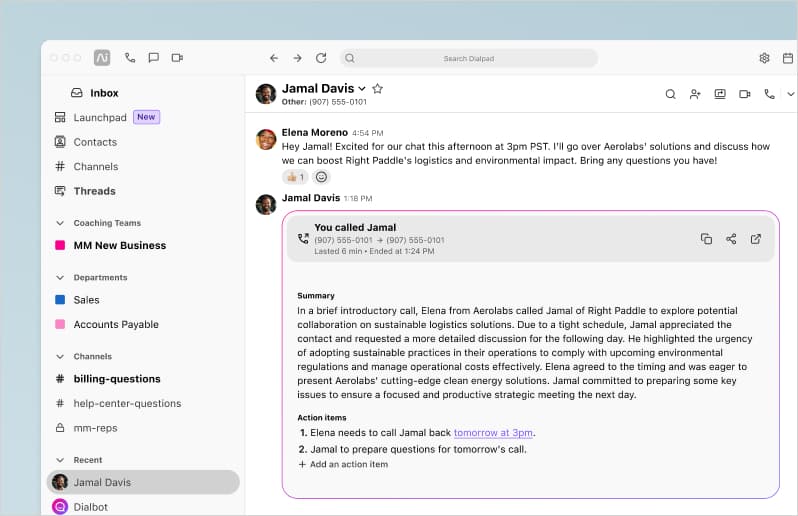
Spot customer satisfaction problems faster than real time with issue detection
As we improve our ability to explain Dialpad CSAT scores, we're using Ai to automatically detect call center issues and generate explanations, including titles, summaries, suggested actions, and counts. This ability to understand a meeting’s topics and scoring is a big deal, but it’s also just the beginning. We’ve already released Ai CSAT, an Ai-calculated customer satisfaction score (a big deal in its own right, given the low survey response rates). But our customers (and their employees actually taking the calls!) want to know why their Ai CSAT is what it is. So our next steps will be providing the dimensions on which scoring is based, tracking event frequency, and finding anomalies, like noticing a certain issue is occurring more often today than it usually does on a Friday. The what of a call is crucial, but to do something about it you also need the why. Ai has the breadth and focus to pull out trends that even the most experienced and diligent managers can’t spot.
Better communication
Business means meeting your customers where they are, whether it’s supporting multiple languages, industry-insider templates, or just the personal touch of
speaking naturally.
Serve a diverse world with multilingual ASR
The world is diverse and multilingual. It’s really important to eliminate obstacles to language switching and language mixing, but to date, it has also been extremely difficult. We have to start by defining a contact center or office's primary language, and if someone speaks in a different language, we’re at risk of returning an error-filled transcript. So we’re working on being able to detect in real time what language is being used in a given call, and switching the ASR (automatic speech recognition) to match. But our long-term goal is truly multilingual transcription, what the industry calls ‘decoding.’ If French goes in, French comes out. If French and English go in, French and English come out. Our tools need to adapt to our habits, not the other way around.
Voice to voice: eliminate lots of IVR middlemen
Here’s how a typical IVR (interactive voice response) system works today: a customer calls to check their account balance. First, ASR gives us a transcript. Then, we use an LLM and other tech to do natural language processing. Next, we do more LLM and workflow work to come up with an answer. Then, we translate that text into speech. Finally, we can play that answer back to the customer. It's an elaborate pipeline and each stage adds delay, which affects how natural the conversation feels. So we’re building a model that does all those intermediate actions by itself: voice goes in, voice comes out. It’s a technologically difficult and computationally expensive challenge. But this kind of end-to-end solution is essential if we want to be able to offer conversations that feel natural as well as useful.
Encourage delightful customer conversations with dialogue templates
At Dialpad we’re fastidious about data and privacy. We’re not just the only business communications stack that develops our Ai in-house; we’re also the only one that gives customers full granular control of which conversations we use to improve their Ai services, and we exclude large amounts of data for reasons like PII (personally identifying information), regulatory compliance, sensitive industries, etc. What remains is a uniquely valuable set of real business conversations which we can break down by sector: transportation, retail, and so on. What we’ve learned from this vast dataset is that customer calls in any given sector tend to be similar and repetitive—people asking about their account balance or the result of their car inspection, over and over. By studying these transcripts, we ought to be able to create general conversational templates. We could start by suggesting questions the agent should ask next to move the conversation through that flow. Next, we’ll look at script adherence; is the agent following the template? Eventually, once we’re confident that these templates accurately represent customer needs, we think we can turn them into chatbot flows.
Advanced tech
Finally, pushing the boundaries of Ai platforms themselves will play a big role in transforming our ability to offer more powerful tools to customers.
LLMs make way for smaller customized models
We’re proud of what we’ve accomplished with DialpadGPT, but we're following a path that will lead to building models that are specific to individual SKUs, industries, companies, even contact centers. We started by rolling out a new feature that lets users add custom dictionary words—a product name, for instance—and that word gets added for everyone who uses that model. Beyond that, we're thinking about domain-specific summaries: you summarize meetings in the legal vertical, for instance, differently from meetings in other contexts. So why not generate a summary and say, ‘By the way, these are the topics we extracted,’ and let the customer request a summary focused on that topic? Ultimately, we want customers to be able to define specific events they want to track with Ai. “I want to see times when the agent says they need more info, and the customer swears and hangs up.” What we have today are simple custom moments, where the customer says, “When these keywords occur in the transcript, do such and such.” But we can imagine more powerful versions.
Deliver more seamless service via on-device Ai
Today we send all our Ai data to powerful cloud-based services which do the analysis and send responses back to the client’s phone or computer. But if you want to reduce latency, you need to perform as much analysis as possible right on the user’s device. Language is one example; you can monitor what language people are speaking and when it changes tell the back end to change the transcription that's being used. We’re seeing this trend on the hardware side: iPhones and Android devices are all getting more circuitry that does Ai processing on-device. Dialpad already has experience doing Ai inference on-device, and the number of processes we handle that way will only grow over time.
Apply Ai to non-linguistic data and features
Last fall we acquired the workforce management (WFM) company Surfboard in order to provide better forecasting, scheduling, and review solutions for customer service teams. The Surfboard system, which is now called Dialpad WFM, does time-series prediction, where you try to estimate staffing levels based on historical data. We’d like to combine numerical data about staffing levels with linguistic analysis of contact center conversations. If you know Fridays are always busy and you’re seeing from the contact center and CSAT scores that there’s a firestorm going on with connectivity issues, you’ll know to staff extra heavily. There’s also lots of implicit data. If we generate a summary and the person instantly shares it with their colleagues, that's a pretty strong signal that they liked it. If we generate a summary and they heavily revise it before sharing it, that's a strong signal in its own right. We want to use this implicit feedback to update our models continuously without our scientists having to take giant data samples and have another crack at the problem.
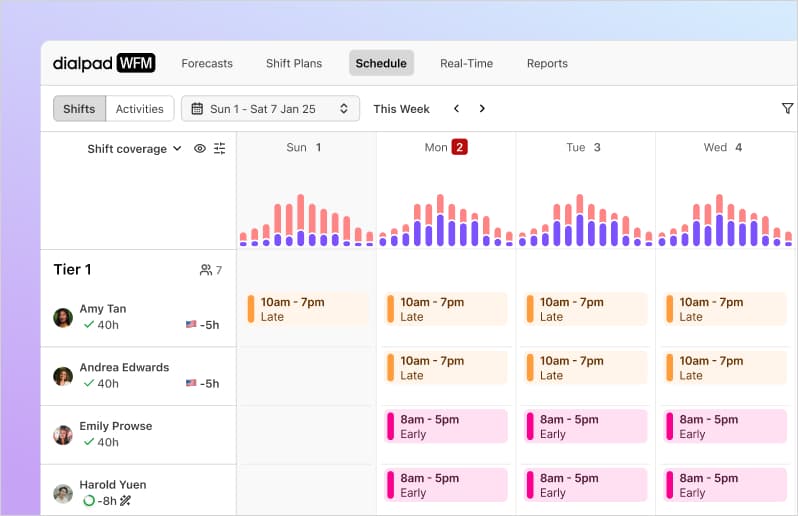
Things move quickly. Let’s all stay tuned.
In the few weeks we spent writing this look at Dialpad’s Ai research and development, both the research and the development have already evolved. The artificial intelligence industry might still be ‘three dogs in a trench coat,’ but those dogs are moving fast, even if they aren’t yet sure where they’re going. So we’ll try to revisit this list later this year, and see how well we foresaw the latest trends in business communication and collaboration.
Maybe we’ll ask DialpadGPT to score it for us. ;)